You have much less privacy than you think you do
- joeeastwood8
- Mar 11, 2021
- 6 min read
Updated: Apr 1, 2021
Increasingly in the current age, decisions about people’s lives are being taken, not based on the people themselves, but on data collected about them. Beyond just targeted advertisements, one needs only recall the A-level exam results fiasco to see why a depersonalised, algorithmic, data-based approach is a terrible solution to many problems (see my previous article on this topic). Amazon faced scandal when it was revealed a secret algorithmic recruiting tool was discriminating against candidates based on their gender [1]. Consider also the social credit system currently being implemented in China where citizens can lose rights and freedoms based on decisions made on the basis of their data [2].
For these reasons data privacy should be a concern to all people and the terrifying truth is we have even less privacy than we think we have. Often, consciously or not, we are trading some of our data for the convenience and features offered in return. However, most of us also have some data which we would not be comfortable sharing publicly and specific apps and companies we would prefer not to share our data with. The difficulty comes when private data can be inferred from public data [3], a problem which through the rapid rise of machine learning and big data has been growing exponentially.
To see a good example of what I mean here simply visit https://adssettings.google.com and you will see a list of categories which Google has sorted you into, such as your age, marital status and educational level. None of this information was explicitly provided by you instead it has been inferred from data which you did provide (Google searches and activity on Google services). For me this list is shockingly accurate. Things can get far more invasive than this, quoting an Oxford university article:
Facebook may be able to infer protected attributes such as sexual orientation, race, as well as political opinions and imminent suicide attempts, while third parties have used Facebook data to decide on the eligibility for loans and infer political stances on abortion.
To crystallise how difficult it is to conceal data while still using a mobile device, let us consider the example of location data. If, for our own reasons, we do not wish our location data to be recorded the solution again seems simple - just disable the GPS location on our phone and the problem is solved. We lose some conveniences normally afforded by collecting this data but gain our privacy, as a result. Well, we mustn't forget that our phone is communicating with cell towers, the locations of which are known. This reduces the privacy we have gained to a rough area within a few kilometres of the tower we are communicating with. Perhaps we are satisfied with our location being known to this low level of precision. If not, we must also disable our mobile data, representing a significant loss in device functionality in the name of privacy.
It is also possible for our location to be deduced from the Wi-Fi networks we connect to. It is common practice in many large distributed networks to correlate connected devices with the location of the Wi-Fi access points they are connected to, when we are logged in to the network our username and email address are also likely connected to this location data. To make matters worse, we don’t even need to connect to the network for our location to be knowable. When we look at our phone, we can see the list of available networks to which we can connect, the only way this list can be built is by constantly sending messages to the access points with information identifying our device (this is called pinging). As we move through the network our location can be deduced as we ping different access points. If the device ID of our phone is static, we can even be tracked over multiple days across multiple networks. This approach of tracking location via Wi-Fi is extremely common and was used by Transport for London in a large-scale investigation into human movement through their stations, it is also common in large campuses such as universities and hospitals. In fact, as many of these institutions use the same network (such as the ubiquity of eduroam at universities) these networks could be used to track people across multiple cities and even multiple countries.
Our only option to guarantee privacy while in these networks then is to deactivate Wi-Fi entirely. Luckily most of the time we are not within these networks so can use Wi-Fi safely knowing our location is now private, right? Here we must take a small tangent to discuss the practice of wardriving. Initially wardriving was the practice of driving around a neighbourhood pinging every Wi-Fi enabled device in range, the idea being that eventually we would encounter an unsecured device and be able to hack or exploit it. This application of wardriving is explicitly nefarious. A more subtle approach is to repeat this process, but to also carry a GPS device. This allows us to triangulate and associate each detected device with a GPS coordinate. If we come back again, we can see which of these devices are static access points such as home routers or smart devices and discard any data which has moved. We now have a map of all the access points in the neighbourhood, scale this up from a small neighbourhood to most large metropolitan areas and suddenly we have an extremely rich dataset. I’m sure you see now where this is going, now when our phone pings any access point anywhere we can cross-reference the device ID against this database and get a reasonable guess of the equivalent GPS location. As before, we don’t even need to be logged in for our location to be detectable.
This is a scary idea in theory, but surely no such dataset exists? Below are two figures, one is a map of Seattle made by undergraduate students in 2004 over several weeks [4]. If this can be achieved in 2004 by a group of students, imagine the masses of data which could be collected today.
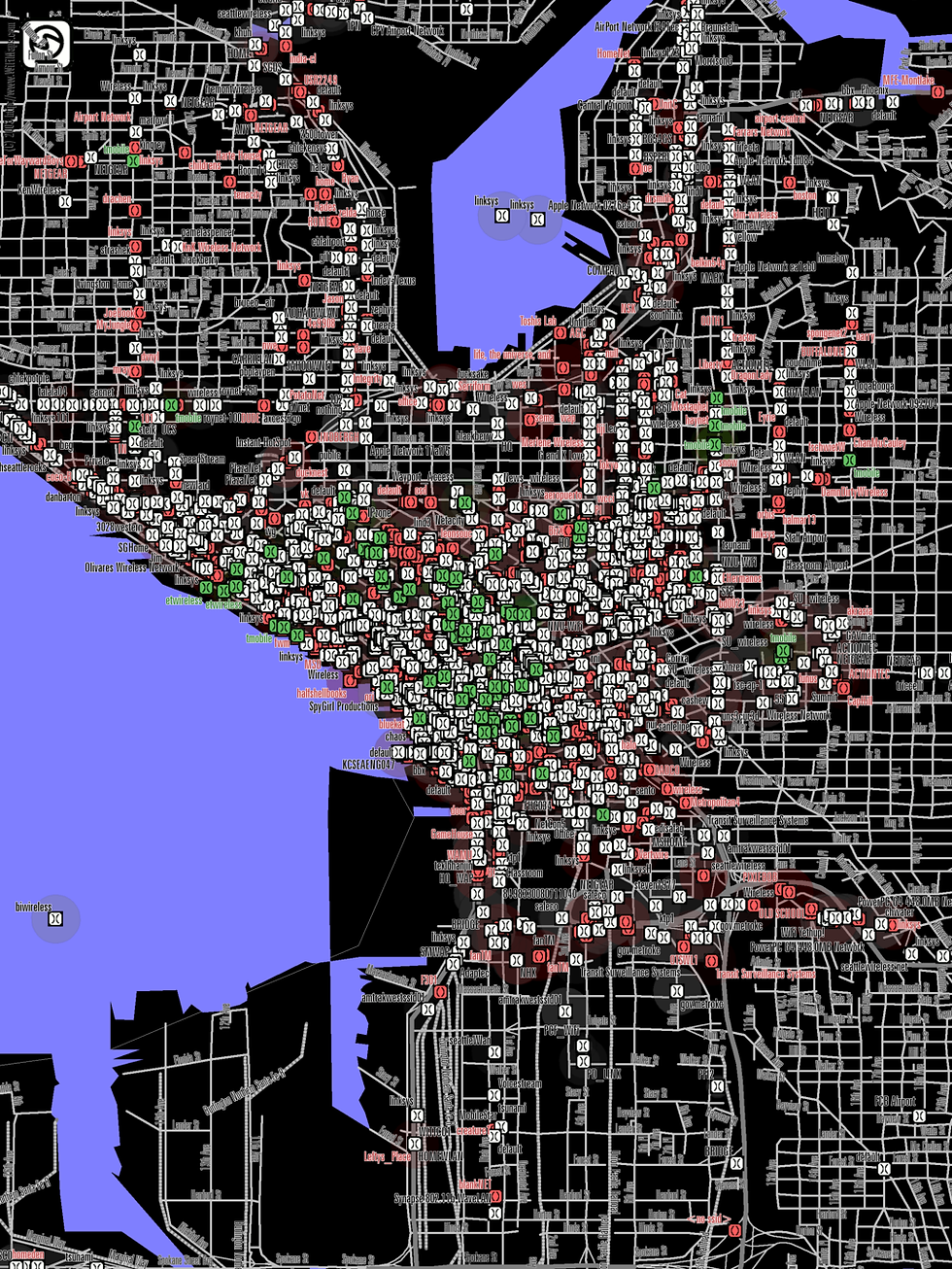
Figure 1: 2004 Map of wireless networks in Seattle
The second shows the WiGLE project which shows all the devices they have mapped across the globe [5].
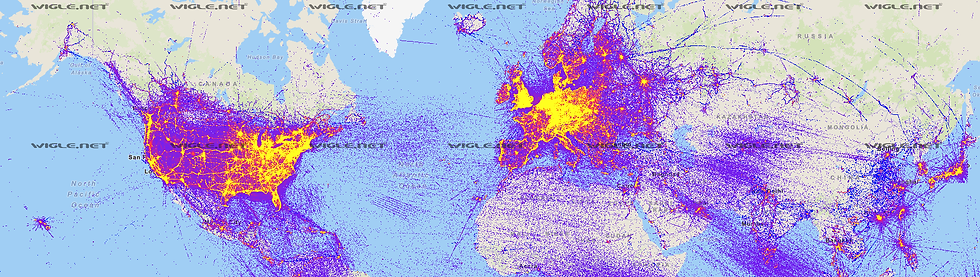
Figure 2: 2021 world map of all mapped networks in the world
While WiGLE is a seemingly positive community driven project, designed to demonstrate the security issues related to wireless networks; many other companies implement similar ideas specifically for providing location data. For example, Skyhook [6] is a company which provides location data for many large companies including Apple and Google – their initial database was entirely constructed via wardriving, they now fuse even more data to provide extremely accurate location estimations.
So now to achieve location privacy we must fully disable Wi-Fi, mobile reception and GPS data, our phone is looking more and more like a useless brick! Yet another route to our location data which has been widely discussed when contact tracing apps were first being deployed is via Bluetooth. Even before the pandemic it was commonplace at large events, such as university open days, for human traffic flow to be monitored by placing Bluetooth ‘beacons’ (which detect when a device enters its signal range and when the device leaves) at strategic locations around the event. Often these beacons' locations are chosen specifically to cover areas where users' phones cannot be traced via Wi-Fi such as outdoor spaces. A network of these beacons can detect a common device ID and, once again, can track the movement of that device through the network.
So, what can be done? As shown above it is not enough to demand that any recorded data be anonymised because high confidence inferences can be drawn to effectively deanonymise the data. The reality is that if even a tiny amount of data is available, with clever analysis conducted over enough people and over enough time, this can constitute a massive loss of privacy. Inevitably, even we do our best to fully remove ourselves from the grid, secondary parties are likely inadvertently collect data which can be related back to us. I predict as processing power and data transfer speeds increase it is likely that photographic data will be the next huge privacy leak, photos are one of the data types we are currently most willing to share but the huge success of facial recognition on image datasets means there is likely a huge amount of data to leverage from these images. We could legislate that the highest standards of data ethics must be followed (anonymising all data before storage, only tracking trends over large populations rather than individuals), such as through the suggested "Right to Reasonable Inferences" [3]; but the only recourse we truly have is to trust that the companies collecting these data do the right thing, and that is a very worrying thought indeed.
Sources
Amazon recruitment algorithm: https://www.reuters.com/article/us-amazon-com-jobs-automation-insight-idUSKCN1MK08G
Social credit: https://algorithmwatch.org/en/chinas-social-credit-system-overdue/
Right to reasonable inferences: https://www.law.ox.ac.uk/business-law-blog/blog/2018/10/right-reasonable-inferences-re-thinking-data-protection-law-age-big
WiGLE: https://wigle.net/
Wardriving and art (contains seattle data, original seemingly removed): https://www.pure.ed.ac.uk/ws/portalfiles/portal/14176763/CREATe_Working_Paper_No_2_v1.0.pdf
Skyhook: https://www.skyhook.com/
The views expressed in blog posts are the views of the author alone and do not necessarily represent the view of Scientists for Labour (SfL) unless posted from the official SfL account.
A solitary Balbir Nagar Escort has the power to convert a night into a lifetime memory with her exquisite erotic methods and tenderly caressing charm. Our registry of talents is the most beautiful, garnering consistent returns from clients rewarded with reliable satisfaction. Do visit our Hi-Fi website and book this ultimate experience.
In Dubai’s fast-paced business environment, having a well-designed website is vital for success. By prioritizing user experience, staying updated with trends, and partnering with experienced professionals, you can create a website that reflects your brand’s values and achieves your goals. Whether you’re a startup or an established business, investing in web design dubai is a step toward digital excellence and long-term growth.
This article highlights how easily our privacy can be compromised without us realizing it. Data collection through simple actions, like Wi-Fi pinging, shows how much we’re unknowingly tracked. It’s a wake-up call to be more mindful of our data. Ghostwriting services UK should consider privacy when handling client data.
if a user frequently engages in sports betting, CBTFExchange might suggest upcoming events, odds, and related games. Similarly, Cbtf Exchange for an RPG enthusiast, the platform could highlight new releases or popular multiplayer campaigns. This level of personalization enhances user satisfaction and loyalty.Gaming is no longer a solitary activity. Platforms like CBTFExchange encourage social interaction by incorporating features such as chatrooms, leaderboards, and multiplayer capabilities. Users can connect with like-minded individuals, share strategies, and compete in friendly matches. This sense of community fosters a deeper connection to the platform and enriches the overall gaming experience.
Selling your house to a cash buyer doesn’t have to be risky. Unlike selling to a traditional buyer, which involves more steps and potential delays, this 7-step guide will show you how to get the best offer and complete the sale as quickly and as safely as possible. Whether you have an immediate need for funds or simply want to offload a house quickly, selling a house for cash can be a great option.
also read: https://homesalehardy.com/